Why Machine Learning is Key to E-commerce Success in the USA
- Elevated Magazines
- Nov 28, 2024
- 10 min read
Are you an e-commerce business owner curious to know about machine learning in e-commerce USA? ML is changing everything. And the USA is directing the way!
According to Statista's report, in 2024, the Machine Learning market is anticipated to attain a value of US$21.14 billion. By 2030, the market volume is anticipated to reach US$134.20 billion, with a compound annual growth rate (CAGR) of 36.07% for the period 2024-2030.
This rapid growth demonstrates the tremendous potential of ML to transform industries and society. Today, you’ll explore why ML is key to success in the USA. At TechVitara, you’ll explore the importance of ML in e-commerce.
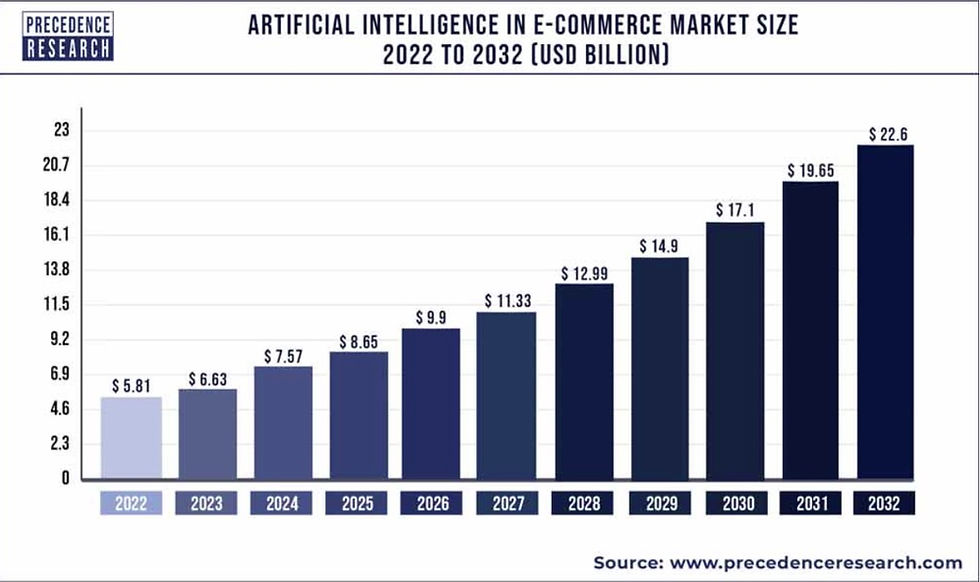
Understanding Machine Learning
Machine learning is a type of artificial intelligence that lets computers learn from data without being instructed. The system improves its capabilities by identifying trends and making decisions. Learning can be either supervised or unsupervised, among other factors. It's used in various fields like healthcare, finance, and technology to make predictions, recognize images, and more. Similar to other sectors, machine learning in e-commerce USA is a current hot topic.
Types of Machine Learning
Have a look at the types of machine learning:
Supervised Learning:
Supervised learning uses labeled data to train models. The algorithm learns by mapping input to the correct output. It is widely used in tasks like classification and regression.
Unsupervised Learning:
Unsupervised learning uses data that has not been identified. The algorithm examines the data for patterns, structures, or connections. It is frequently used to group data, identify outliers, and reduce the number of dimensions.
Reinforcement Learning:
With reinforcement learning, models are taught by giving them prizes and punishments. Through interacting with its surroundings, the agent learns how to make choices. This learning is common in games, robots, and other autonomous systems.
The E-commerce Landscape in the USA
The USA e-commerce landscape is super competitive. Business giants like Amazon and Walmart are driving the e-commerce market forward. Mobile shopping, personalized shopping, and fast delivery services are all growing areas of e-commerce.
Growth of E-commerce in the USA
In the USA, e-commerce has evidenced immense growth. This growth became more evident in the past ten years. Facilities such as smartphones, worldwide internet access, and digital payment boosted it further.
Furthermore, the COVID-19 outbreak accelerated internet buying. It does not matter if it is electronics or food, people these days prefer to buy things online. Speed and convenience are the key factors. Additionally, machine learning in e-commerce USA boosted the overall growth.
Key Players in the U.S. E-commerce Market
Amazon
Amazon is the largest e-commerce company in the U.S.
It offers everything from books to electronics, clothing, and groceries.
Amazon Prime, its subscription service, provides faster shipping and exclusive deals.
Walmart
Walmart is a retail giant with a strong online presence.
It offers a variety of products, including groceries, electronics, and home goods.
eBay
eBay is known for its online auction and consumer-to-consumer sales.
It also allows businesses to sell directly to consumers.
eBay offers a wide range of products, including collectibles and electronics.
Shopify
Shopify is a platform that helps organizations create their online stores.
It provides tools for marketing, payments, and shipping.
Many small and large businesses in the U.S. use Shopify for e-commerce.
Target
Target is a major retailer with a growing e-commerce platform.
It offers numerous products, including fashion, home goods, and groceries.
Target offers same-day delivery through its Shipt service.
Key Players | Description | Notable Offerings |
Amazon | Largest e-commerce company in the U.S., offering a wide variety of products. | Amazon Prime (faster shipping, exclusive deals). |
Walmart | Retail giant with a strong online presence, selling groceries, electronics, and home goods. | Wide range of products, competitive prices. |
eBay | Known for online auctions and consumer-to-consumer sales. | Auction platform, collectibles, electronics. |
Shopify | E-commerce platform helping businesses create online stores. | Tools for marketing, payments, and shipping. |
Target | Major retailer with a growing e-commerce platform, offering fashion, home goods, and groceries. | Same-day delivery through Shipt service. |
Current Challenges Faced by E-commerce Businesses
Below are the current challenges faced by E-commerce Businesses:
High Competition: E-commerce is growing, leading to more businesses online. It is hard to stand out and attract customers.
Customer Expectations: Shoppers expect fast delivery, easy returns, and 24/7 customer support. Meeting these high standards can be challenging.
Logistics and Shipping Issues: Handling shipping, delivery delays, and managing returns can be costly and complex for businesses.
Cybersecurity Threats: E-commerce sites face hacking attempts, data breaches, and fraud. Protecting customer data is critical but challenging.
Changing Technology: E-commerce platforms constantly need updates. Keeping up with the latest technology requires money and expertise.
Payment Fraud: Online businesses often deal with fraudulent transactions, fake payments, and chargebacks, which affect profits.
The Role of Machine Learning in E-commerce
Machine learning in e-commerce USA is revolutionizing e-commerce. ML helps businesses run more efficiently. It gives customers a better experience. Take a look at three crucial areas where ML is making a big difference:
Enhancing Customer Experience
Personalization Techniques:
ML thoroughly studies user data. This analysis assists ML in providing personalized shopping experiences.
Customized recommendations are offered. This recommendation are based on browsing history, past purchases, and preferences.
Recommendation Systems:
ML-driven systems suggest relevant products, boosting user engagement and conversions.
Examples include "customers also bought" or "recommended for you" sections.
Inventory Management Optimization
Demand Forecasting:
Machine learning forecasts future sales trends based on historical data and market parameters.
Assists businesses in optimizing stock levels. It avoids overstocking or stockouts.
Supply Chain Management:
ML enhances logistics and delivery efficiency. It boosts delivery by analyzing supply chain data.
Improves delivery timings, reduces operational costs, and prevents delays.
Pricing Strategies and Optimization
Dynamic Pricing Models:
ML adjusts real-time prices based on demand, customer behavior, and competitor actions.
Ensures pricing is competitive while increasing profits.
Competitor Price Monitoring:
Machine learning tracks competitors’ prices, allowing businesses to stay competitive in real time.
Helps optimize pricing strategies. It balances market competitiveness with profit margins.
Data-Driven Decision Making
In today's e-commerce landscape, data-driven decision-making is essential to stay competitive. Data assists businesses in understanding customer behavior. Moreover, it helps forecast trends and optimize operations.
Businesses boost sales and minimize risk by making data-based decisions integrating machine learning in e-commerce USA.
Importance of Data in E-commerce
Data is what makes e-commerce work. Every sale, customer interaction, or website click generates valuable data.
Properly analyzed data reveals numerous information, such as:
What do people want?
How do they shop?
How well do items operate?
E-commerce sites use these to improve their marketing. Moreover, it boosts inventory management and user experience.
For example, businesses can recommend customers about products that would be good for them based on what they have bought before. Plus, it keeps people coming back, which means more sales.
How Machine Learning Analyzes Data
Machine learning in e-commerce USA is changing how e-commerce looks at their data. Machine learning algorithms can swiftly sift through massive volumes of data. This analysis identifies patterns and trends that may not be obvious at first. E-commerce enterprises may make informed decisions by automating data analysis. It enhances procedures and generates more revenue.
Predictive Analytics
Predictive analytics examines data to forecast the future. In e-commerce, it assists businesses in predicting how customers will behave.
Customers' behavior, such as which goods will be popular at various seasons of the year. Moreover, this analytics also identifies potential client turnover concerns.
With this information, businesses can adjust their marketing strategy. Furthermore, companies may optimize their inventory utilization and provide targeted incentives to keep customers holding.
Sentiment Analysis
Sentiment analysis is a machine learning in e-commerce USA method that uses consumer input, such as reviews or social media comments. This analysis determines how people feel about something (positive, negative, or neutral).
Businesses that sell things online utilize this to determine how satisfied their consumers are. Moreover, it gives an idea of how they might improve their products or services based on reviews. Businesses can remain flexible and meet customer demands. This real-time feedback loop is very beneficial for e-commerce.
Marketing Strategies Enhanced by Machine Learning
Machine learning (ML) introduced businesses to new ways to market their products and services. It allows them to work more efficiently and achieve better outcomes. Here are some significant ways that ML improves marketing:
Targeted Advertising
Precision: ML algorithms analyze massive quantities of data to better target groups and ensure that adverts reach the intended audience.
Dynamic Ad Creation: Machine learning can automatically generate advertising based on user choices, enhancing engagement rates.
Real-time Optimization: ML models can make real-time changes to campaigns based on performance data, which increases ROI.
Customer Segmentation
Behavioral Analysis: ML algorithms segment customers based on purchasing behavior, preferences, and interactions, enabling personalized marketing strategies.
Predictive Segmentation: ML predicts which segments are likely to convert by analyzing historical data. It facilitates targeted campaigns.
Enhanced Insights: Businesses can uncover hidden patterns in customer data, leading to more effective segmentation strategies.
Enhancing SEO Strategies
Keyword Optimization:
Data-Driven Insights: ML tools analyze search trends and user behavior to identify high-performing keywords.
Competitive Analysis: Businesses can assess competitors’ keyword strategies and adjust their own for better visibility.
Content Personalization:
User Preferences: ML analyzes user data to tailor content recommendations, improving consumer engagement and retention.
Content Quality: Algorithms evaluate content performance, helping marketers create more relevant and appealing material.
Fraud Detection and Risk Management in E-commerce
E-commerce security is essential for trust. Moreover, it ensures transitions run efficiently. With the immense rise of e-commerce, fraud has become increasingly common. Here are some of the essential points:
Importance of Security in E-commerce
Establishing trust: Customers need assurance that their personal and financial information is safe.
Preventing Financial Loss: Fraud can lead to significant losses for businesses and consumers.
Regulatory Compliance: Many countries have laws requiring strong data protection measures.
Machine Learning Techniques for Fraud Detection
Machine learning in e-commerce US transforms the way businesses detect and prevent fraud. Some effective techniques include:
Anomaly Detection: It identifies any unusual pattern in traction data. Such transactions could indicate fraud.
Classification Algorithms: These algorithms, like decision trees and neural networks, help classify transactions as legitimate or fraudulent.
Natural Language Processing (NLP): It analyses customer communications for sins of potential fraud.
Reducing Cart Abandonment through Predictive Analysis
An issue that comes up a lot in e-commerce is cart loss. This problem can be solved with predictive analysis by:
Identifying At-Risk Customers: Businesses can find customers likely to abandon their carts by looking at their behavior.
Personalized Engagement: Customers may be more likely to finish their purchases if you send them focused reminders or discounts.
Improving User Experience: Customers find it easier to complete their purchases when they get a streamlined checkout procedure.
Case Studies of Successful Implementation
Case Study 1: Amazon
Amazon Music leverages Amazon SageMaker with NVIDIA GPUs to optimize machine learning (ML) training and inference. This approach enhances search performance while reducing costs, allowing users to discover music and podcasts more efficiently.
Case Study 2: Walmart
Walmart leverages AI & ML in supply chain management through automation, contract negotiations, and customer interactions, achieving efficiency, cost savings, and innovation while addressing challenges in trust and technological agility.
Case Study 3: eBay
eBay leverages AI & ML across its platform to enhance personalized shopping experiences, streamline seller success, and optimize marketplace efficiency, driving innovation and creating a more engaging, accessible global e-commerce environment.
Challenges and Limitations of Machine Learning in E-commerce
Machine learning (ML) offers great potential for e-commerce, but it faces several challenges and limitations. Have a look at the possible challenges with machine learning in e-commerce USA:
Data Privacy Concerns
Customer Data Security: Collecting data raises privacy issues. Customers worry about how their information is used.
Regulatory Compliance: E-commerce businesses must comply with laws like GDPR. It adds complexity and costs.
Integration with Existing Systems
Legacy Systems: Many e-commerce platforms use outdated systems. Integrating ML can be challenging.
Cost of Implementation: Upgrading systems to accommodate ML can be expensive and time-consuming.
Dependence on Quality Data
Data Quality: ML algorithms need high-quality data. Poor data can lead to inaccurate predictions.
Data Bias: If the data is biased, the ML models will also be biased. This can harm customer trust and business decisions.
Future Trends of Machine Learning in E-commerce
Emerging Technologies
Machine learning in e-commerce is evolving with personalized recommendations, AI-driven chatbots, predictive analytics, and enhanced customer experience for seamless shopping.
Feature | Description |
Customization | Machine learning will make shopping more unique by personalizing the experience for each consumer. |
Chatbots | AI-driven chatbots will provide instant, on-the-spot solutions to customer queries and concerns. |
Visual Search | Consumers will utilize visual search features, allowing them to search for products using images instead of text or voice. |
Recommendation Systems | Advanced algorithms will analyze user behavior to suggest goods, offering highly relevant product recommendations. |
Voice Commerce | Voice commerce features will enhance the shopping experience, enabling consumers to shop hands-free through voice commands. |
Augmented Reality | Augmented reality (AR) will allow consumers to virtually try on clothing and visualize how it looks, improving confidence in appearance before buying. |
Predictions for the Next Five Years
More automation: The business operational cost will decline as artificial intelligence will automate more tasks.
Businesses will better understand their clients by gathering information on them.
Frauds: Machine learning will make it easier to detect and prevent fraud.
Supply Chain Optimization: Artificial intelligence will streamline managing, supplying, and transferring items.
Focus on Sustainability: Businesses will utilize ML to decrease waste and implement sustainable practices.
Better security: Machine learning will strengthen security solutions.
Conclusion
In conclusion, machine learning in the USA is evolving the e-commerce landscape. It improves customer experience and simplifies supply management. It's growing better at implementing personalized marketing strategies.
As technology continues to develop, additional innovative concepts will emerge. ML and artificial intelligence with predictive capabilities will rule the future. Companies that want to succeed in the contemporary digital economy must adapt to change with time.
FAQs:
What is the significance of machine learning in e-commerce?
Machine learning improves decision-making, automates processes, and enhances personalization, making it essential for optimizing pricing, product recommendations, and marketing strategies in e-commerce.
How does machine learning enhance customer experience?
Machine learning helps customize product recommendations, improve search results, and offer personalized promotions, leading to a seamless, relevant, and engaging shopping experience for customers.
What are the challenges of implementing machine learning in e-commerce?
Challenges include data privacy concerns, high costs, technical expertise, and integrating machine learning models with existing e-commerce platforms effectively and securely.
Can small businesses benefit from machine learning in e-commerce?
Yes, small businesses can use affordable machine learning tools to enhance customer insights, optimize marketing, personalized shopping experiences, and automate tasks, boosting competitiveness.
What are the future prospects for machine learning in the e-commerce industry?
Machine learning will continue to evolve, with future trends including smarter personalization, voice commerce, enhanced visual search, and better fraud detection, transforming e-commerce landscapes.